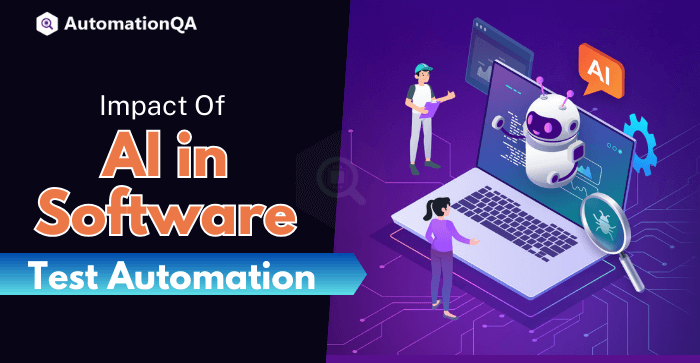
In today’s digital landscape, users’ expectations are skyrocketing. Satisfying these expectations requires reliable and flawless software, which is only possible through a robust software testing process. As development cycles shorten and the pressure to release high-quality software intensifies, traditional testing methods often fall short. Manual testing is not only slow, but it also requires careful attention as it is susceptible to error. In contrast, automated testing can need help keeping pace with the sheer volume and variety of test cases necessary for modern applications.
It is precisely where Artificial intelligence comes into the picture. This groundbreaking technology has transformed the entire software validation process. AI addresses the common challenges of the test automation process by significantly enhancing efficiency, accuracy, and speed. It offers intelligent solutions that may anticipate possible problems before they become serious, learn from historical data, and adjust to new testing automation situations. But, despite its transformative potential, integrating AI into quality assurance has challenges.
In this blog, we’ll explore why AI is becoming an indispensable tool for the modern software validation process.
AI-driven Software Test Automation
AI fundamentally transforms testing dynamics by introducing speed, accuracy, and efficiency.
AI-powered systems can examine large datasets to build test cases and automatically detect potential problems. Thus, AI streamlines testing workflows and enhances overall reliability.
Another significant advantage of integrating AI is that it revolutionizes automation testing. It not only simplifies and accelerates the testing process, but it also ensures its accuracy. AI-powered systems can examine large datasets to build test cases and automatically detect potential problems.
Moreover, AI continuously utilizes machine learning algorithms to improve test case quality based on past testing experiences. It enables testing teams to achieve broader coverage, minimize errors, and optimize testing costs.
Significance Of AI In Software Testing
Integrating AI into testing automation is crucial for revolutionizing testing practices. AI-driven automation enhances test coverage, expedites test execution, and identifies intricate patterns that may elude human testers. By intelligently analyzing data and adapting to evolving software test automation environments, AI empowers testing to be more comprehensive, efficient, and adaptive. Ultimately, this leads to delivering higher-quality software products within shorter timeframes, meeting the demands of today’s rapidly evolving technology landscape.
Efficiency and Resource Optimization
Developers often need more resources and time to conduct exhaustive testing manually. AI-powered automation can intelligently identify areas suitable for automation based on repetitive patterns, optimizing time, human resources, and capital.
Faster Deployments
With developers aiming for faster deployments amid inadequate infrastructure, AI-driven automation offers a suitable solution to streamline testing processes and expedite software releases.
Cost Reduction
Automating repetitive testing tasks, which constitute 80% of testing efforts, helps the test automation company to reduce costs and effort associated with manual testing. AI automation efficiently handles repetitive tasks, allowing human testers to focus on innovative and exceptional test scenarios.
Enhanced Testing Capabilities
Combining human intelligence with AI automation enables the recognition of application issues in innovative test environments. By leveraging AI algorithms, testers can focus on critical aspects while leaving repetitive tasks to automation.
Improved Productivity
AI algorithms, such as machine learning and deep learning, can optimize testing processes and enhance productivity by identifying areas for optimization and streamlining testing workflows.
Increased Bug Detection
AI-driven automation facilitates the detection of a maximum number of bugs in less time, leading to more reliable and accurate software applications. Testing automation companies can refine products based on test outcomes and learn from trial and error. So, AI in testing offers a more innovative, efficient, and productive approach to software evaluation, ultimately benefiting developers and end-users.
Benefits Of Using AI In Software Testing
Harnessing AI within your testing protocols can enhance your efficiency and efficacy. Let’s explore the core advantages of artificial intelligence in software evaluation.
Enhanced Test Coverage and Quality Assurance
Integrating AI bolsters test coverage by identifying gaps and ensuring comprehensive examination. AI analyzes extensive datasets to pinpoint elusive bugs, enhancing software quality for test automation consulting companies. For instance, generative AI autonomously generates test cases based on software specifications, broadening coverage and improving testing quality by identifying recurring issues and patterns.
Complementary Role of Manual Testing
AI augments manual testing efforts by automating repetitive tasks, freeing up manual testers to focus on exploratory testing and critical thinking. By automating mundane tasks, AI enhances testing efficiency, allowing skilled testers to delve deeper into software functionalities and uncover potential edge cases.
Time Efficiency
AI-driven automation accelerates the testing cycle without compromising accuracy. Automated tests can be swiftly executed, covering multiple scenarios simultaneously round-the-clock. Additionally, AI expedites test data generation for automation testing companies, ensuring thorough testing under various conditions and resulting in quicker feedback loops, facilitating early bug detection and resolution.
Reduced Time-to-Market
By streamlining testing processes and accelerating test cycles, AI reduces time to market. Parallel testing capabilities and early bug detection enable faster bug fixing and optimization, which is crucial for ensuring timely product releases without sacrificing quality.
Enhanced Accuracy
AI-powered testing tools deliver unparalleled precision and consistency, minimizing the risk of human error. Whether executing regression tests or testing complex algorithms, AI ensures reliable and accurate results, which is critical for maintaining software integrity and reliability.
Real-Time Monitoring
AI facilitates real-time monitoring in software testng by analyzing vast datasets and identifying subtle deviations from expected behavior. AI uses machine learning algorithms to quickly identify anomalies, allowing for proactive problem-solving before users are impacted. AI-powered monitoring also aids in predicting potential issues based on historical data, ensuring uninterrupted software performance.
Effective Risk Management
AI assists in risk assessment by simulating real-world scenarios and uncovering vulnerabilities early in the development cycle. AI identifies potential security breaches and performance bottlenecks by subjecting software to diverse usage patterns and attack simulations, enabling proactive mitigation measures.
Streamlined Test Environment Management
AI-driven automation revolutionizes test environment management by swiftly provisioning required environments and effortlessly managing environment variations. It ensures accurate and consistent automation testing by populating test environments with relevant data, improving the accuracy of test results.
Future Trends For Software Testing 2024
Increased Demand for Low-Code Testing Platforms
- Organizations increasingly turn to low-code testing platforms to streamline testing processes amidst growing complexity.
- With Agile and DevOps methodologies dominating the industry, the need for rapid development and deployment has surged, driving the adoption of low-code testing platforms to accelerate testing cycles while ensuring reliability.
- Furthermore, the global shortage of skilled testers has increased the demand for solutions that enable non-technical users to participate in software test automation activities. Thus making low-code testing platforms indispensable assets in the software evaluation process.
Increased Focus on Shift-Left
- Organizations are strategically shifting automated testing activities in the development pipeline.
- Shift-left testing can help teams collaborate more effectively between development and testing. It assists companies in lowering the expenses and labor needed for remediation at a later stage of the development cycle. Additionally, it helps deliver more reliable software products to the market.
Web Accessibility Testing
- There is a notable shift towards prioritizing web accessibility testing, driven by the increasing importance of aligning with Web Content Accessibility Guidelines (WCAG) and global regulations.
- Regulatory organizations worldwide are imposing rigorous requirements on testing automation firms to ensure that web applications are accessible to users with varying abilities. Consequently, throughout the development lifecycle, software teams prioritize accessibility considerations.
AI/ML Integration in Testing
- AI and machine learning (ML) integration will become increasingly popular.
- AI and ML technologies can transform testing, including test case creation, fault identification, analysis, and strategy optimization.
Test Environment Simulation & Green Computing
- Organizations are leveraging service virtualization to simulate hardware resources and reduce energy consumption in data centers, contributing to environmental sustainability and cost savings.
- Service virtualization enables more efficient computing resource use and reduces energy waste for a test automation company. Thus aligning its digital operations with environmental responsibilities.
Breaking Down Silos
- There is a growing trend towards breaking down silos between development and QA teams, fostering cross-functional testing teams to drive quality throughout the software development lifecycle.
- Cross-functional testing teams support industry trends toward innovation and collaboration by accelerating feedback loops, identifying and resolving difficulties early in the process, and delivering superior products to market.
Final Words
The impact of AI in software examination is profound, with advancements, applications, and future trends shaping the quality assurance landscape. From enhancing predictive defect analysis to revolutionizing test environment management, AI drives accuracy and sustainability for test automation consulting firms. Thanks to developments in AI-powered testing solutions, organizations may now achieve higher test coverage, faster testing cycles, and faster release of more dependable software products to market. Moreover, AI applications extend beyond traditional boundaries, encompassing web accessibility testing, low-code testing platforms, and cross-functional testing teams. AI and machine learning use in software testing is expected to increase, emphasizing strategy optimization.
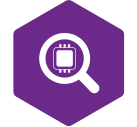
AutomationQA
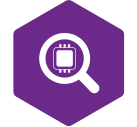
Latest posts by AutomationQA (see all)
- Cypress Cloud Meets AI: The Future of Automated Testing - February 18, 2025
- End-to-End Testing with Cypress: Tips for Faster and More Reliable Tests - February 11, 2025
- Everything you need to know about Selenium Grid: Large-Scale Test Automation - February 7, 2025