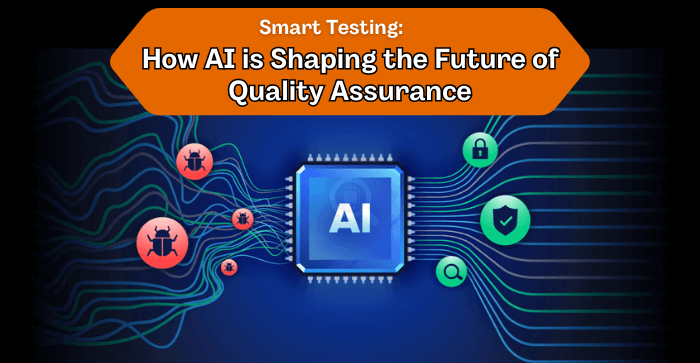
Gone are the days of monthly or bi-monthly software and mobile app releases. In today’s fast-paced world, businesses must ensure quick releases to stay competitive. Traditional software testing methods are of no use here. They are slow and prone to human mistakes. Therefore, software companies need a quick, reliable, and accurate solution to keep pace with these frequent build releases. This is precisely where AI steps in and revolutionizes the entire testing domain.
AI-driven tools reduce the time required to identify and resolve defects. This way, these tools accelerate testing and improve market time. Further, they automate various repetitive tasks, improving testing accuracy and consistency. These tools also help share data and deliver valuable customer support. Therefore, around 63% of companies implement it worldwide in their quality assurance process. But if you are still trying to figure out the practicality of AI and wonder how it can help you in QA, continue reading this blog.
Role Of Artificial Intelligence In QA
AI makes QA more efficient by reducing testing time. It expedites the release process while guaranteeing comprehensive test coverage, giving companies a competitive edge and a faster time to market. Organizations use AI tools for image-based, continuous, and API testing. AI further helps testers create, execute, and analyze test cases, reducing their need for manual updates. Overall, AI helps in innovation and upscaling testing services to give them a strategic edge in the market.
Five Steps For Implementing Artificial Intelligence In QA
Step 1: Organizational Readiness And Defining Objectives
Before integrating AI into the testing process, you must clearly understand your testing scope and objectives. I have a detailed understanding of your testing procedures and the instrument for this. Check whether they are feasible for AI integration or not. Next, decide what testing to do and what to achieve. Find out the areas where integrating AI can speed up the process. Remember to select the software testing tools and methods based on the project scope and AI models,
Step2: Investing In Training
When you have selected the appropriate tool for your implementation process, it’s time to train your QA team to use it. Your training section must elaborate on and cover the tool’s technical aspects, including functionality. Only in this way can you ensure maximum and optimum utilization of this tool. You must also encourage hands-on experience through smaller projects, as it helps build confidence and understand its practical uses.
Step 3: Integrating AI
Finally, it’s time to integrate AI-based software testing into your testing process. Remember, this is a very critical step; therefore, it has to be done gradually. You must start the integration process with less critical projects and then expand it to more vital areas. This faced approach helps in building confidence among your team. They become more adoptable and learn more about this tool, essential for a smoother transition.
Step 4: Monitor and optimization
Simply integrating AI tools with traditional testing methods will not solve the problem. To maximize its benefits, you must monitor progress. Continuously monitoring its performance helps you identify areas that need improvement. For better test automation optimization and more accurate results, collecting feedback from your team, including your stakeholders, is essential.
Step 5: Data quality and security
Undoubtedly, AI can handle complex test cases and automate various repetitive tasks. However, it also relies on data and training to generate accurate and high-quality outcomes. Therefore, remember that your AI models will need regular retraining with high-quality test data. This keeps the AI model current, ensures quality results, and improves accuracy.
Benefits Of Integrating AI In Software Testing
Improved Accuracy
Even the best testers can make mistakes during repetitive manual testing. However, AI-integrated automated testing can perform the same steps correctly every time, ensuring accurate results in less time and allowing testers to focus on creating new tests and handling complex features.
Beyond Manual Testing Limitations
Manual testing can’t realistically simulate a web application test with thousands of users. AI-enabled testing can simulate hundreds or thousands of virtual users interacting with applications, networks, or software.
Benefits for Developers and Testers
Developers can use shared automated tests to catch issues early in the development cycle. Further, AI-enabled tests inform automation testing companies about the changes in the source code by alerting them whenever a test fails. This boosts confidence among the team and also saves their precious time.
Increased Test Coverage
AI allows for deeper and broader test coverage to improve software quality. It can check memory, file contents, internal states, and data tables to ensure the software meets the requirements. AI can run thousands of test cases to provide coverage that manual testing can’t match.
Time and Cost Savings
Repeating manual tests with each source code change is a time-consuming and expensive. AI can help a test automation company automatically generate a suite of test cases. Further, NL can convert the test requirements directly into the executable test script. This reduces testing time from days to hours, directly translating into cost savings and faster time to market.
Predictive Analysis
AI can analyze customer data to understand user needs and browsing habits. It allows testers, designers, and developers to anticipate user standards and offer better support. Machine learning further improves the predictions based on real-time user behavior.
Enhanced Writing of Test Cases
AI improves the quality of test cases for testing automation companies. It offers realistic test scenarios that are simple to control and run quickly. AI enables developers to analyze project data and figure out new approaches to test cases in seconds.
Improved Regression Testing
Fast deployment increases the need for regression testing, which can be overwhelming when done manually. AI, along with ML, reduces the complexity of the regression testing. AI can also scan for UI changes, such as color, shape, or size, and validate these changes, which would otherwise require manual testing.
Visual User Interface Testing
AI enhances user interface design and visual approval of web pages. It allows automation testing companies to test different UI contents that typically need human intervention. Machine learning-based visualization technologies can identify minor photo variations that people may miss.
Enhanced Defect Tracing
AI can quickly and automatically trace bugs and errors, preventing future hindrances. The quantity of flaws rises with the growth of data and software. AI quickly identifies these bugs and duplicates, allowing the development team to operate smoothly and efficiently. AI-based bug tracking perceives duplicate errors and identifies failure fingerprints, improving overall defect management.
Best Practices For Implementing AI In Software Testing
Research AI Tools
Investigate and select the best-suited tools before incorporating AI into testing. Many AI-powered tools are available, each with advantages and disadvantages. Popular AI-powered tools include Selenium, Testim, Katalon Studio, and Applitools. Evaluate these tools to determine which best meets your requirements.
Develop a Test Strategy
Creating a comprehensive test strategy is crucial when incorporating AI into your testing process. The organization can accomplish this by providing the team with unobstructed communication channels. This further guarantees that everyone on the team understands the objectives and standards, ensuring that the testing services are well-prepared for AI integration.
Promotes Collaborations
Effective team collaboration is essential for the maximum utilization of AI in the testing process. The organization can accomplish this by providing the team with unobstructed communication channels. This further guarantees that everyone in the team understands the standards, goals, and objectives. Companies must also encourage open dialogue among team members to share insights and feedback. By cooperating, businesses can identify issues before the development process.
Outcome
AI-based software testing is redefining the landscape of QA. Organizations may improve their testing procedures and make them more scalable and efficient by adding AI. Deeper insights, potential problem prediction, and repetitive work automation are all provided by AI solutions, freeing up testers to concentrate on more complicated cases. It leads to faster release cycles and the delivery of higher-quality products. As digital transformation accelerates, the adoption of AI in QA becomes essential. By producing superior products, businesses can gain a competitive edge, streamline operations, and save costs. Fusing AI with QA ensures agility, precision, and continuous improvement. As a result, using AI in testing is now essential rather than a futuristic idea. AI will be a significant factor in determining how software testing develops in the future since it will spur innovation and quality in the field as technology advances.
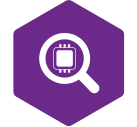
AutomationQA
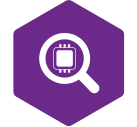
Latest posts by AutomationQA (see all)
- Cypress in Action: Enhancing Software Testing in Modern Development Practices - October 18, 2024
- Achieving QA Excellence By Implementing Continuous Test Automation for Faster, Better Software - October 10, 2024
- Mastering Mobile App Testing With Top Selenium Best Practices - October 3, 2024